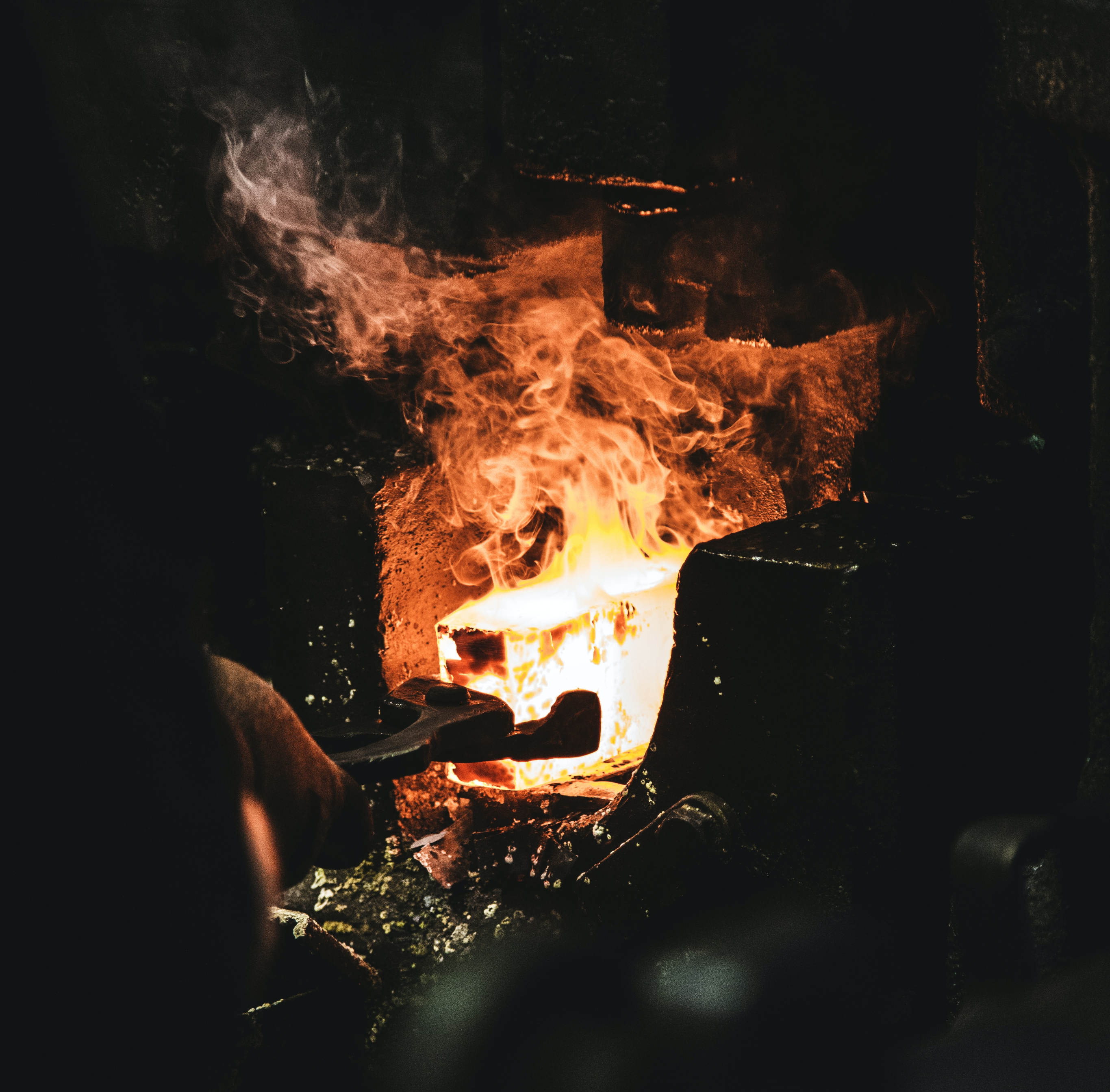
The steel market is divided into different segments based on form, production methods, and end-user industries. In terms of form, the market is categorised into liquid steel, crude steel, and finished steel. In terms of production, the market is classified into blast furnace-basic oxygen furnaces (BF-BOF), electric arc furnaces, and other methods. The end-user industry segment includes automotive and transportation, building and construction, tools and machinery, energy, consumer goods, and other industries.
In 2021, the Steel Market Size reached a value of USD 1307.9 billion. The steel industry is expected to experience substantial growth, with projections indicating a rise from USD 1353.28 billion in 2022 to USD 1718.26 billion by 2030. This represents a compound annual growth rate (CAGR) of 3.47% during the forecast period spanning from 2022 to 2030. The market's expansion is primarily attributed to the increased demand for steel resulting from growing infrastructural activities in both commercial and residential construction sectors. Additionally, the availability of domestic raw materials such as iron ore and a cost-effective labour force contribute significantly to driving the market's growth.
Steel remains the backbone of modern infrastructure and manufacturing, underpinning countless industries globally. The steel market is segmented based on forms (liquid steel, crude steel, finished steel), production methods (blast furnace-basic oxygen furnaces [BF-BOF], electric arc furnaces, and others), and end-user industries (automotive, construction, tools, energy, consumer goods, and more).
In 2021, the global steel market reached a valuation of USD 1307.9 billion, with projections to grow at a CAGR of 3.47%, hitting USD 1718.26 billion by 2030. Key drivers of this expansion include booming infrastructure projects in residential and commercial sectors, coupled with accessible domestic raw materials like iron ore and a cost-effective labor pool.
India has emerged as the world’s second-largest producer of crude steel, with a production volume of 120 million tons in FY2022, up from 102.5 million tons in FY2021. This growth is supported by abundant iron ore reserves (India ranks fifth globally) and competitive labor costs. The government’s initiatives, like the Pradhan Mantri Awas Yojna and the 100 Smart Cities Mission, are fueling demand, with the construction sector alone accounting for 40% of India’s steel consumption in FY2021.
Despite these achievements, challenges persist. Limited investment in R&D and insufficient design and engineering capabilities made India a net importer of steel in FY2019. However, the sector bounced back, becoming a net exporter by late FY2020.
The steel industry in India faces hurdles like:
Addressing these issues requires embracing digitization and adopting innovative solutions.
The steel industry stands at the cusp of a technological revolution, with digitization offering unprecedented opportunities. Technologies like the Internet of Things (IoT), big data analytics, artificial intelligence (AI), machine learning (ML), and cloud computing are driving efficiency, productivity, and sustainability.
Artificial Intelligence (AI) and Machine Learning (ML) play a crucial role in achieving digitization within the steel industry. The integration of AI and ML technologies offers numerous benefits and opportunities for transforming various aspects of the industry. Firstly, AI and ML can enhance the automation of processes, enabling the steel sector to streamline operations, reduce manual intervention, and improve overall efficiency. By leveraging AI algorithms, the industry can optimize production schedules, predict maintenance requirements, and minimise downtime by identifying potential issues before they escalate. ML algorithms can analyse vast amounts of historical and real-time data to identify patterns, trends, and anomalies, facilitating data-driven decision-making and enabling continuous process improvement.
In India, steel manufacturing companies are at varying stages of their digital transformation journeys. While some firms have successfully implemented AI technologies to optimise production processes and reduce losses, others are actively exploring ways to enhance business intelligence through the utilisation of AI and analytics.
1. Predictive Maintenance: The steel industry can use AI and ML for predictive maintenance by analysing real-time data from equipment sensors. This helps detect potential failures, allowing proactive maintenance scheduling and optimising production efficiency. For example, Blast Furnaces can be monitored using thermal imaging to identify hot spots and prevent damage to the equipment. This technology predicts the health of various equipment components without disrupting production.
2. Quality Control: AI and ML algorithms analyse production and quality data to improve product quality. Steel manufacturers can implement data-driven quality control measures to ensure consistent and high-quality output. For instance, in the case of SBM (Special Bar Mill), a rolling mill for producing rounds, maintaining quality is crucial. By using industry-grade cameras and a deep learning model, defects like scratch marks and dents can be identified in real-time. This allows for timely corrective actions, preventing a large number of quality issues.
3. Inventory Management: AI algorithms can analyse historical demand patterns, market trends, and other relevant factors to provide accurate demand forecasting. This enables steel companies to optimise inventory levels, reduce carrying costs, and avoid stockouts or excess inventory, improving overall supply chain efficiency. AI algorithms are used for inventory management. By analysing historical demand patterns, market trends, and other relevant factors, these algorithms provide accurate demand forecasting. This helps optimise inventory levels, reduce carrying costs, and prevent stock outs or excess inventory. As a result, overall supply chain efficiency improves, benefiting steel companies by reducing costs and ensuring timely delivery of products.
4. Energy Management: ML algorithms analyse energy consumption patterns to optimise energy usage in steel manufacturing. This reduces waste, lowers costs, and promotes sustainability. For instance, a steel plant can deploy sensors throughout the facility to monitor energy consumption, temperature levels, and sound patterns. ML algorithms trained on historical data learn to recognize patterns and correlations between sensor data and maintenance needs. Real-time analysis helps identify impending maintenance states, such as unusual energy consumption, abnormal temperature rises, or irregular sounds indicating machinery issues. The algorithms generate maintenance alerts, enabling proactive measures to be taken by plant operators.
5. Supply Chain Optimization: AI and ML optimise the steel industry's supply chain, improving production planning, logistics, and procurement. By analysing historical data and market trends, these technologies enhance efficiency, reduce lead times, and improve coordination. For instance, demand forecasting models optimise production and procurement based on accurate predictions. Intelligent inventory management systems reduce carrying costs and ensure timely order fulfilment. Collaborative relationships with suppliers enhance visibility and responsiveness. Transportation management systems and route optimization algorithms minimise costs and delivery times. By analysing data and implementing continuous improvement initiatives, companies identify bottlenecks, enhance customer service, increase agility, and adopt sustainable practices.
6. Safety and Risk Management: AI and ML improve safety and risk management in the steel industry by analysing sensor data and historical records. These technologies identify hazards, predict incidents, and enable proactive measures for a safer working environment. For example, computer vision techniques can be used with a small camera to detect objects and promptly alert operators to potential collisions with workers. Another application is monitoring compliance with safety regulations using CCTV feeds and deep learning models. Real-time analysis generates alerts for non-compliance, enabling immediate corrective actions. These examples demonstrate how intelligent cameras and continuous monitoring enhance safety measures by leveraging AI and ML technologies.
Overall, the adoption of AI and ML technologies in the steel industry holds immense potential for enhancing productivity, efficiency, quality control, supply chain management, and sustainability efforts. By leveraging these technologies, steel companies can stay competitive in the market and drive continuous improvement in their operations.
The adoption of AI in steel production is not without challenges. Key issues include:
Despite these barriers, the slow adoption of AI in the steel industry represents an opportunity. Small-scale experiments and an openness to change can lead to transformative results.
Digitization, powered by AI and ML, is reshaping the steel industry. Indian steel manufacturers are at various stages of this transformation, from optimizing production to enhancing business intelligence. Companies like JSW Steel, Tata Steel, and SAIL are already exploring these technologies, setting benchmarks for innovation.
Key drivers of this transformation include:
At Nwarch.AI (a subsidiary of Daten and Wissen), we are proud to collaborate with industries on their digitization journeys. By harnessing technologies like computer vision, deep learning, and AI-driven analytics, we empower businesses to:
Our mission is to support the steel sector and beyond in overcoming challenges and unlocking their full potential. Whether it’s predictive maintenance, supply chain optimization, or safety enhancements, we provide cutting-edge solutions tailored to industry needs.
Partnering for Digital Transformation: Nwarch.AI's Role in Driving Innovation Across Industries
We, at Nwarch.AI (a subsidiary of Daten and Wissen), collaborate with numerous industries to support their digitization journeys and achieve remarkable outcomes. Through our expertise in computer vision, deep learning, and data-driven solutions, we empower organisations to enhance productivity, promote sustainability, and ensure a safer working environment. By leveraging advanced technologies, we enable businesses to tackle challenges, optimise operations, and drive positive transformations in their respective domains.
Written by
Daten & Wissen is the team of expert AI engineers to help your business to embark on a transformational journey with the adoption of this futuristic technology.
Video surveillance systems hold a hidden treasure trove of valuable data that remains l…Read more
This website uses cookies to improve your experience. We'll assume you're ok with this, but you can back-out if you wish.